scikit_decorators¶
Module: missions.nodes.regression.scikit_decorators
¶
Scikit decorators for optimizing hyperparameters
Inheritance diagram for pySPACE.missions.nodes.regression.scikit_decorators
:
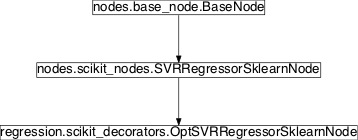
OptSVRRegressorSklearnNode
¶
-
class
pySPACE.missions.nodes.regression.scikit_decorators.
OptSVRRegressorSklearnNode
(C=1, epsilon=0.1, kernel='rbf', degree=3, gamma='auto', coef0=0.0, shrinking=True, tol=0.001, verbose=False, max_iter=-1, **kwargs)[source]¶ Bases:
pySPACE.missions.nodes.scikit_nodes.SVRRegressorSklearnNode
Decorator wrapper around SVRRegressorSklearnNode
Epsilon-Support Vector Regression.
This node has been automatically generated by wrapping the sklearn.svm.classes.SVR class from the
sklearn
library. The wrapped instance can be accessed through thescikit_alg
attribute.The free parameters in the model are C and epsilon.
The implementation is based on libsvm.
Read more in the User Guide.
Parameters
- C : float, optional (default=1.0)
- Penalty parameter C of the error term.
- epsilon : float, optional (default=0.1)
- Epsilon in the epsilon-SVR model. It specifies the epsilon-tube within which no penalty is associated in the training loss function with points predicted within a distance epsilon from the actual value.
- kernel : string, optional (default=’rbf’)
- Specifies the kernel type to be used in the algorithm. It must be one of ‘linear’, ‘poly’, ‘rbf’, ‘sigmoid’, ‘precomputed’ or a callable. If none is given, ‘rbf’ will be used. If a callable is given it is used to precompute the kernel matrix.
- degree : int, optional (default=3)
- Degree of the polynomial kernel function (‘poly’). Ignored by all other kernels.
- gamma : float, optional (default=’auto’)
- Kernel coefficient for ‘rbf’, ‘poly’ and ‘sigmoid’. If gamma is ‘auto’ then 1/n_features will be used instead.
- coef0 : float, optional (default=0.0)
- Independent term in kernel function. It is only significant in ‘poly’ and ‘sigmoid’.
- shrinking : boolean, optional (default=True)
- Whether to use the shrinking heuristic.
- tol : float, optional (default=1e-3)
- Tolerance for stopping criterion.
- cache_size : float, optional
- Specify the size of the kernel cache (in MB).
- verbose : bool, default: False
- Enable verbose output. Note that this setting takes advantage of a per-process runtime setting in libsvm that, if enabled, may not work properly in a multithreaded context.
- max_iter : int, optional (default=-1)
- Hard limit on iterations within solver, or -1 for no limit.
Attributes
support_
: array-like, shape = [n_SV]- Indices of support vectors.
support_vectors_
: array-like, shape = [nSV, n_features]- Support vectors.
dual_coef_
: array, shape = [1, n_SV]- Coefficients of the support vector in the decision function.
coef_
: array, shape = [1, n_features]Weights assigned to the features (coefficients in the primal problem). This is only available in the case of a linear kernel.
coef_ is readonly property derived from dual_coef_ and support_vectors_.
intercept_
: array, shape = [1]- Constants in decision function.
Examples
>>> from sklearn.svm import SVR >>> import numpy as np >>> n_samples, n_features = 10, 5 >>> np.random.seed(0) >>> y = np.random.randn(n_samples) >>> X = np.random.randn(n_samples, n_features) >>> clf = SVR(C=1.0, epsilon=0.2) >>> clf.fit(X, y) SVR(C=1.0, cache_size=200, coef0=0.0, degree=3, epsilon=0.2, gamma='auto', kernel='rbf', max_iter=-1, shrinking=True, tol=0.001, verbose=False)
See also
- NuSVR
- Support Vector Machine for regression implemented using libsvm using a parameter to control the number of support vectors.
- LinearSVR
- Scalable Linear Support Vector Machine for regression implemented using liblinear.
POSSIBLE NODE NAMES: - OptSVRRegressorSklearnNode
- OptSVRRegressorSklearn
POSSIBLE INPUT TYPES: - FeatureVector
-
__init__
(C=1, epsilon=0.1, kernel='rbf', degree=3, gamma='auto', coef0=0.0, shrinking=True, tol=0.001, verbose=False, max_iter=-1, **kwargs)[source]¶
-
__hyperparameters
= set([ChoiceParameter<kernel>, LogUniformParameter<C>, NoOptimizationParameter<input_dim>, NoOptimizationParameter<dtype>, NoOptimizationParameter<output_dim>, QLogUniformParameter<max_iter>, NoOptimizationParameter<retrain>, NoOptimizationParameter<store>, NoOptimizationParameter<kwargs_warning>, LogNormalParameter<epsilon>, NoOptimizationParameter<cache_size>, NoOptimizationParameter<shrinking>, LogUniformParameter<gamma>, NoOptimizationParameter<verbose>])¶